How many touchdown passes can Tom Brady be expected to throw in a given game this season?
The New England Patriots quarterback, who is heading into the new NFL season with a contract extension still officially unresolved, averaged 1.75 touchdown passes last season. But in more than half of his games he threw for one touchdown or fewer. Which statistic is more important for evaluating his success?
Mean and median are both measures of what statisticians call central tendency. The mean is a measure of the average value of all of the data points in a sample. The median is the value for which half of the data points are above that value and the other half are below it. Both have their uses in evaluating a set of numbers, but both also have weaknesses.
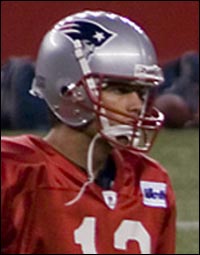
The 2009 Season
The Brady example is useful for illustrating the pros and cons of the two statistics.
He threw 28 touchdown passes over 16 games last season, for mean (or average) of 1.75 touchdowns per game. However, with three games in which he threw no touchdown passes, and six in which he threw just one, his median touchdown passes per game was one (in this case, half of the points are greater than or equal to one and half are less than or equal to one).
On the one hand, it might seem that the mean statistic is a more fair representation of Brady's performance for the season. What usually gets talked about is how many touchdowns a quarterback threw in a given year, suggesting more interest in the mean statistic. Indeed, the mean statistic can be seen as the expected value of the number of touchdown passes Brady would throw in a given game -- or the number of touchdown passes he would throw in probability in a given game -- and it shows that there is a good chance that he will throw more than one touchdown pass.
On the other hand, a different take on the season might lead you to a different conclusion. Brady threw zero or one touchdown passes in more than half of his games in the 2009 season, and only threw more than two touchdowns four times, and only once against a team that finished better than .500 (Indianapolis). The other three times - Tennessee, Tampa Bay and Jacksonville -- did not have final scores closer than 28 points, including a 59-0 rout of Tennessee that included six Brady touchdowns. These games greatly helped Brady's average, but may not accurately reflect the season he had. (Figures from espn.com)
This is not to say that one measure is necessarily better or worse. Rather, it highlights the fact that both emphasize certain aspects of the season.
Looking at the median statistic essentially ignores the fact that Brady had four games with three or more touchdown passes. You would certainly prefer a quarterback who had four big games to one who didn't have any. But looking at the mean may over-emphasize the big games. Because of the fact that Brady's six touchdowns against Tennessee amounted to an extreme value, that game altered the average more than two of the games in which he had no touchdowns.
While both mean and median are used frequently, the mean may be the most common. Since it is an unbiased estimate of expected value, it has a predictive value that is easily used both formally by statisticians and informally by people every day. From points per game, to grade-point averages to miles per gallon, we take for granted that averages are a good standard of performance and predictor of things to come.
One of the main criticisms of using mean statistics is that they place too large an emphasis on extreme values, such as Brady's big game against Tennessee. Since very large or very small values affect an average more than values near the middle, it can be argued that mean statistics skew our perception of central tendency.
The median statistic has some nice properties in this respect, as it is largely unaffected by outliers and therefore may be a more reasonable portrayal of the experiences of most people in measures such as income.
Off the Gridiron
Some economists emphasize the usefulness of median statistics to measure income. Doing so, they say, gives a better picture of more people's living conditions by using traditional per capita income measures to avoid giving undue weight to those at the top of the income spectrum.
However, there are drawbacks to this approach. In addition to losing the advantages of the nice statistical properties of means, using the median statistic minimizes the importance of extreme values in the income spectrum, and it may be that an added weight to those values is desired. In the median income example, it could be argued that using median income paints an economic picture that is perhaps grimmer than it actually is, as the fact that people have a chance to obtain high incomes is arguably downplayed.
Just as it was with Tom Brady in 2009, there are several high values at the top of the income distribution. It is difficult to decide which is a more appropriate income measure because using the mean statistic puts a greater emphasis on the high values than may be appropriate, and may not do as good of a job of reflecting the common experience, while using the median doesn't take into account the positive effects of having some people with very high incomes. The difficulty in judging which measure to use or pay more attention to is that both highlight information we care about, but the information is slightly different between the two measures.
Arthur Smith is a recent graduate of Georgetown University, with a degree in international economics. He spent his junior year studying at the London School of Economics. At the State of the USA, he has worked on education and economy projects, including, the data selection, collection and presentation processes. He has also worked as a research assistant on projects analyzing returns on education, and perceptions of AIDS in Kenya.